Dropping the copilot: Keeping track of AI assistants can be a tricky business
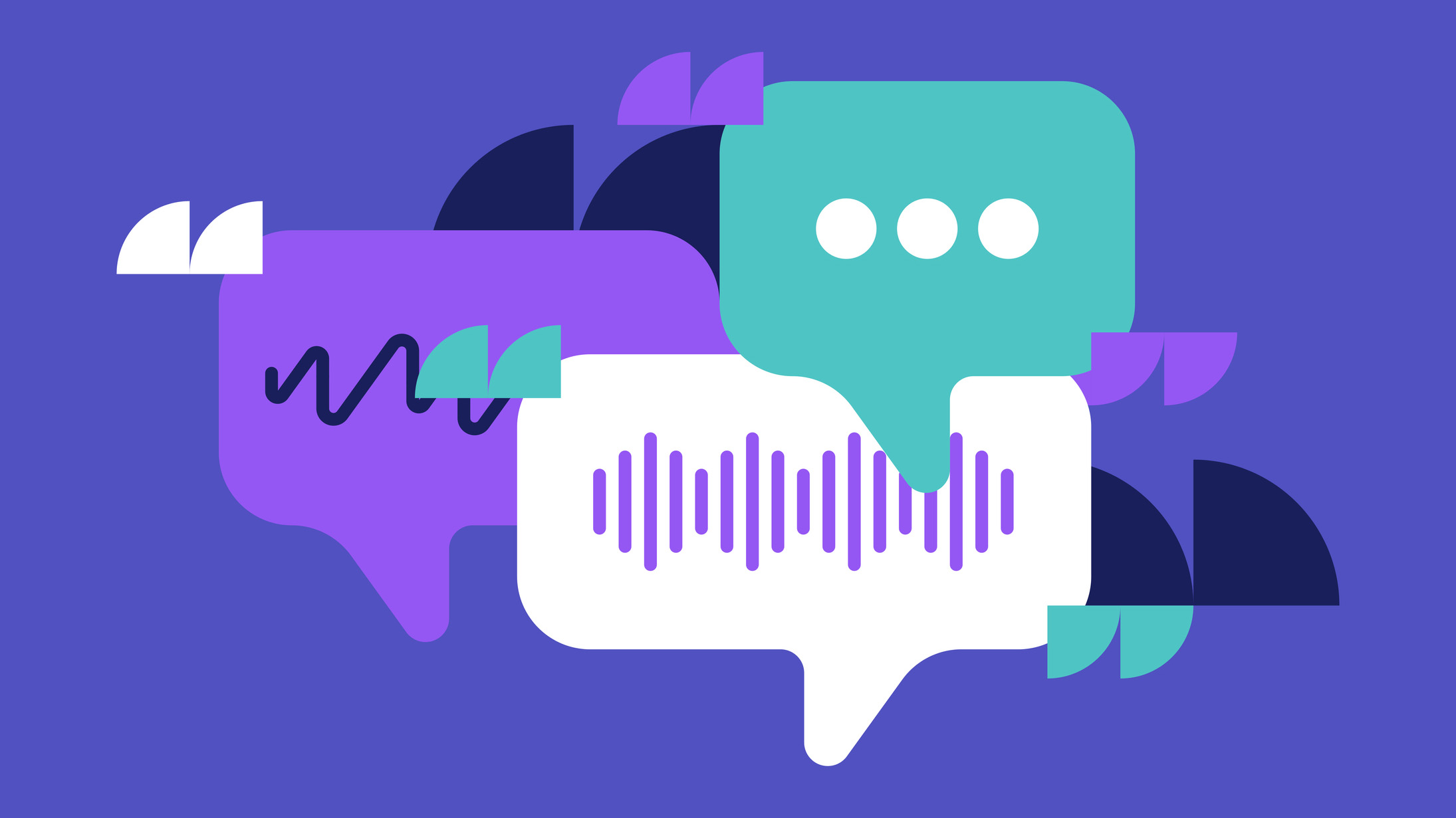
Anyone who has a Microsoft 365 subscription will have noticed Copilot popping up when you create a new document, and offering to help. Or if you use Google Workspace you’ll be used to Gemini offering to summarize emails and automate writing in Docs.
These, though, are just some of the most visible examples of how generative AI is spreading across the enterprise, especially among knowledge workers.
Almost all vendors are adding some form of AI capability, whether it is chatbots, copilots or enhanced search to their applications. AI can remove backgrounds in photos, create summaries of Zoom calls, and suggest the best way to configure a firewall’s security settings. The promise is that these AI tools will save time and hopefully, money.
Too many helpers
AI tools can, of course, save time. As with any form of automation, often they are at their best when it comes to repetitive tasks such as answering FAQs. These are also tasks where humans often make errors, especially if they are working for long periods of time. Chatbots don’t need to take a break.
They are also good at searching through large volumes of documents or other unstructured data, and producing summaries.
And large language models (LLMs) make searching easier, because they work in natural language and can process data in the context of wider organizational knowledge or policies. You no longer have to know about Boolean search terms or “Google dorking” to find useful results. Gemini, or Copilot within Microsoft Bing, takes care of that.
Chatbots have also found a niche in public-facing web applications, where they can filter customer service queries and provide product recommendations. There have been public problems too, with AI assistants and chatbots sometimes returning inappropriate suggestions.
But an even bigger issue for enterprises could be the sheer proliferation of AI tools across their applications.
AI tools are now included in productivity applications, such as Microsoft 365 and Google Workspace, as well as other “packaged” business applications, enterprise software and SaaS. Each has their own approach to AI, use different models and training data, and take different approaches to handling queries.
As Dale Vile, CEO at industry analysts Freeform Dynamics suggests, organizations can find themselves wrestling with multiple AI-enabled assistants, potentially linked to competing generative AI vendors.
At best this will produce inconsistent results. At worst, it risks undermining confidence in AI, and in the business’ efforts to create their own tailored, secure and confidential AI tools.
“It is a bit of a mess,” he tells ITPro. “There is lots of redundancy and overlap.” And research by Freeform Dynamics found that over a third of CIOs that had deployed Microsoft Copilot were disappointed with it.
Then there are the costs: embedded AI tools are, typically, not free. The cost of everyone in the business switching them on, whether they need them or not, quickly adds up.
“With SaaS vendors trying to charge an amount per user per month [for AI], you would pay a fortune if you switched on all the AI elements,” Vale adds.
Balancing AI reliance
The rapid development of generative AI over the last couple of years leaves businesses trying to balance the productivity gains offered by the technology, with the need for accuracy, security, confidentiality and an effective user experience.
Larger enterprises can develop the capability to build their own AI systems, linking large language models to retrieval augmented generation (RAG) and to their own data sources. “They can build AI infrastructure for their own applications, just as they would build any infrastructure,” says Vile.
The alternative is to use third party, web-based AI tools. As Vile suggests, this gives organizations a greater degree of control than relying on software vendors’ own AI offerings; at the very least it allows businesses to standardise on one LLM, and develop prompts, guardrails and other rules to govern how it is used.
But it also allows businesses to tailor AI tools to their own requirements, and design AI so that it helps solve that organisation’s problems. “You have to know what you are trying to achieve,” he says.
Clarity about how AI will be used focuses the investment, and avoids some of the pitfalls around confidentiality and security.
“You do have to get away from thinking of AI as an abstract, Swiss army knife of capabilities,” Vile suggests. “You have to be very clear about what you are trying to do and how these tools will help you along the way.” As with any IT project, sitting down with users and discussing their workflows, and how they can be improved, will go a long way to making the technology work.
Making AI work for you
Some of the strongest AI use cases Freeform Dynamics has seen are where teams use the technology in a tightly focused way.
Creative teams use AI to speed up the production process, and to automate steps such as removing background noise from videos. In software development, AI is helping junior coders, and teams responsible for maintaining applications. Vile points to teams using AI to audit code, and to create documentation. “It’s picking off specific problems,” he says.
Within his own firm, Vile uses Anthropic’s Claude and Perplexity on a daily basis. These are assistants that help teams but which stay firmly under the analyst’s control.
Tasks include visualization, drafting specific documents such as client proposals, and even virtual “personas” of IT industry stakeholders that serve as a sounding board for research questions.
In some cases, these tools increase productivity by as much as 50%, and easily repay the time spent on creating the right prompts and workflows. “It’s tuning how you work with each model,” he says.
Simply letting employees use embedded AI tools is far less likely to be effective, Vile cautions. Businesses need to take a structured approach to avoid losing oversight of employee AI use and allowing shadow AI to proliferate. And he goes as far as to suggest that employees should not use tools such as Copilot until they have been trained.
Rather than just turning on AI, businesses should start with specific use cases and build up knowledge and experience. Otherwise, embedded AI tools could be a risky, and expensive, distraction.
Source link