The history – and future – of automation in security
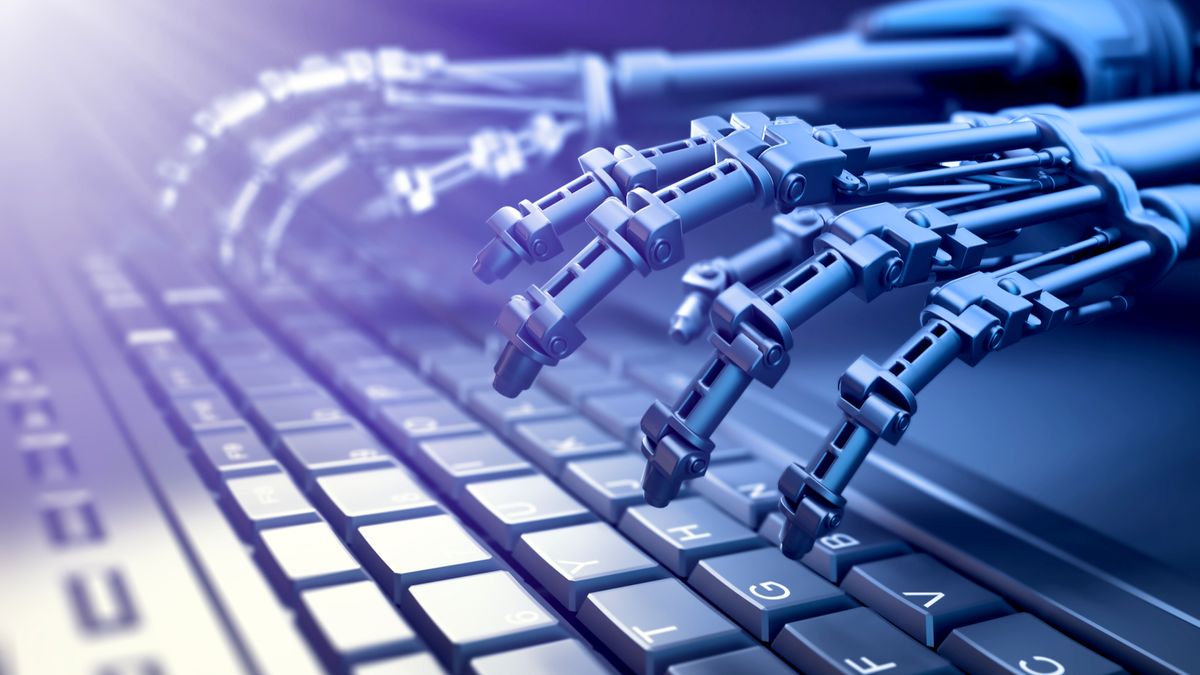
As cyber threats continue to grow in volume and frequency, the role of automation in the security sector has never been more critical. Over the previous four decades automation has evolved from rudimentary log management to holistic, AI-powered threat detection and response capabilities that adapt to the current threat landscape as it evolves.
This development has fundamentally transformed how organizations defend themselves against cyber attacks, enabling faster responses, reducing human error, and improving the overall security posture of millions of enterprises around the world.
This article will explore the history and future of automation in the security sector, detailing how the industry has progressively automated an increasing share of the security tasks required for enterprises to maintain a robust cyber posture.
Automation’s early beginnings in the security sector
The security industry began its automation journey in the early 1980s with automatic log management, generating basic alerts to help security professionals manage an ever-increasing number of alerts.
As security software became more mature and widely adopted among businesses, enterprise IT infrastructure complexified significantly. This made analyzing the vast amounts of log data coming back from the various devices on the network impossible for small security teams composed of a few analysts. As a result, businesses were able to reduce the amount of leg work their analysts would need to perform by implementing basic automation for log management workflows. But this was only the first step in a long journey of automation for the sector.
During the late 1980s and early 1990s some security vendors went further than their pioneering predecessors and developed tools to actually help detect potential attacks as they happen, offering the first iterations of the intrusion detection system (IDS), which are now commonplace in the industry today.
An IDS automates the process of detecting unauthorized access on the network, looking out for signs of malicious activity before alerting the security operations center (SOC) to take action.
In its earliest guises, IDS solutions were primarily signature-based, meaning they needed to have been given exactly what constitutes malicious activity. The result was that security professionals would need to manually update the system on the latest threats in order to keep their organization protected. As such, the earliest iterations of IDS tools, although certainly helpful, were fairly easy to circumvent for experienced cybercriminals.
Moving into the 2000s and automation in security took a leap forward with the introduction of security information and event management (SIEM) tools. During this period the technology sector was going through a period of rapid growth, and along with it came a flood of new software tools companies could use to keep their IT estate safe.
One drawback to this explosion of security innovation was that the amount of data these tools generated exploded too, and SIEMs were a vital step in helping security staff manage this data overload.
SIEMs helped to automate the correlation of logs and alerts across a wide variety of sources, making the process of detection far more efficient. Around the same time these tools were hitting the market, businesses also began looking into automating their responses to threats as well.
It’s all well and good to have a centralized system collecting all the alerts and flagging potential threats but this is not much use if the attack happens during the middle of the night when nobody is on hand to take action.
As a result, automated incident response (IR) became the next step in the automation of the security sector. Automated IR tools allowed security teams to write predefined rules and workflows that could investigate and remediate potential attacks with minimal, or absolutely no human intervention.
Machine learning revolutionizes automation
During the 2010s automation took a leap forward as software companies began integrating machine learning (ML) algorithms into their products.
No longer relying on static signature detection, ML algorithms meant firms could detect new threats without having to explicitly instruct the system on what constitutes an attack.
This drastically improved their ability to detect anomalies and identify threats in real-time, as the algorithms would slowly evolve alongside the threat landscape to reflect the latest attack vectors and TTPs.
Traditional signature-based threat detection systems were plagued by a high rate of false positives, where legitimate activity was mistakenly flagged as a potential attack.
ML algorithms avoid making the same mistakes by learning from historical data to form a picture of what constitutes normal user behavior on the network, otherwise known as a baseline assessment
This helps security tools distinguish between normal and malicious activity on the network, and saves security teams from wasting time investigating harmless activity that was flagged as suspicious.
As AI has become more widespread and the software leveraging it more sophisticated, it has become something of a minimum requirement for security vendors.
The latest solutions use a combination of generative and more traditional statistical AI to automate even more security tasks, such as exposure management, deleting duplicate credentials, revoking access of offboarded employees, patching vulnerabilities as they appear, and updating software.
Firms will look to invest in consolidating automated processes in the future
Enterprises are not slowing down when it comes to embracing automation to improve their cyber resilience. A recent survey of 300 IT and security professionals, conducted by Sapio Research on behalf of automation platform Seemplicity, found 97% of respondents had already invested in some level of automation.
Moreover, data collected by Wakefield Research in 2023 showed that 80% of organizations had concrete plans to increase their investments in cybersecurity automation through 2024.
The benefits of automating security workflows have been clear to see for some time now, as demonstrated in IBM’s 2020 Cost of Downtime report, which found security automation cut breach costs in half.
“Companies studied who had fully deployed security automation technologies (which leverage AI, analytics and automated orchestration to identify and respond to security events) experienced less than half the data breach costs compared to those who didn’t have these tools deployed – $2.45 million vs. $6.03 million on average,” IBM found.
Despite a strong appetite for automation across enterprises, the results of the Seemplicity study revealed that just under half (44%) of IT professionals still rely on manual methods to some extent when managing security workloads, showing firms still have some way to go before they reach a fully autonomous cyber defense.
It also found the primary areas of focus for the next wave of investments in automation were vulnerability scanning (65%), vulnerability prioritization (65%), and remediation processes (41%).
As automation creeps into every area of cybersecurity the next step for the industry is to consolidate the various branches of automation highlighted above into a unified platform that provides a more cohesive and efficient way to manage these processes.
In a report on the future of SOC automation platforms, Francis Odum, cybersecurity researcher, and independent analyst, and Josh Trup, an early-growth investor at Greenfield Partners, outlined this vision.
“We believe security automation tools will leverage their deep integrations into the existing security stack and rich historical workflow data to become the data fabric for which the future SOC AI Assistants are built, transforming struggling units into proactive powerhouses.”
These automated platforms will help ensure businesses can take full advantage of the automating capabilities of technologies like AI while ensuring humans are kept in the loop when necessary.
Source link