“There needs to be an order of magnitude more effort”: AI security experts call for focused evaluation of frontier models and agentic systems
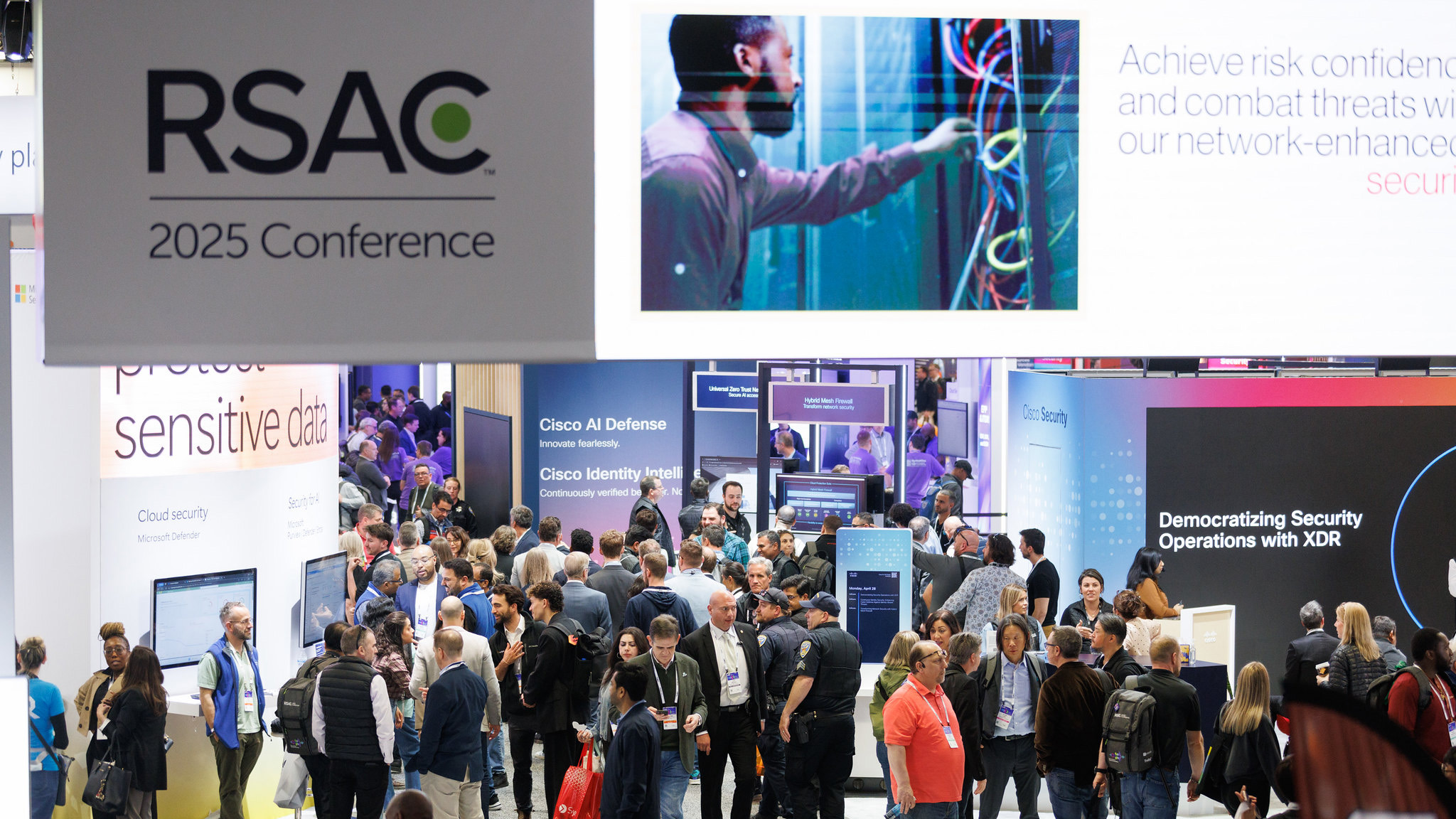
Much more detailed work must be done to evaluate the security and safety risks associated with adopting AI models, according to a panel of experts in the field.
At RSAC Conference 2025, representatives from Google DeepMind, Nvidia, and the UK AI Security Institute emphasized the current challenges involved with evaluating AI model risks and the uphill challenge security teams face to keep up with the rapidly-evolving nature of AI agents and complex AI systems.
Jade Leung, CTO at the UK AI Security Institute, said there is still a lot of open questions on the potential risks of agentic AI systems, with safety and security assessments currently unable to keep pace with the rapid development of AI systems.
Leung said that while many AI companies are working on adopting dangerous capability evaluations, it’s a very hard process to do well and the extent to which it’s an “evolving science” is underappreciated right now.
“We totally do see companies make substantial investments and build substantial teams, that are really running super hard at the problem,” Leung said.
“And we see companies who haven’t quite got teams are staffed up yet for a variety of reasons,” Leung added, declining to give a specific example when prompted by Ram Shankar Siva Kumar, data cowboy at Microsoft and panel host.
“I think some companies are taking a really good stab at it, I think there needs to be an order of magnitude more effort on it really.”
Daniel Rohrer, VP of Software Product Security, Architecture & Research at Nvidia argued that as AI systems become more complex, organizations will need to shift to evaluating entire AI systems.
He explained the likes of agentic AI and mixture of experts models are harder to assess from a security perspective, necessitating continuous to ensure organizations can still predict the behavior of the systems they’ve deployed.
“A lot of people are like, ‘Oh well, this model did this horrible thing’ – well, it’s meant to be general purpose, it’s meant to do 3,000 things, for the system I need it to just do one and I can force it to do that one very specifically and very narrowly.”
“And that control, especially when we start thinking about AGI and others, that ability to exert control as complexity rises, as autonomy rises, is going to be really important.”
John ‘Four’ Flynn, VP of Security and Privacy at Google DeepMind, agreed with the notion that security teams must repeatedly revisit the behavior of models and systems. He stated that AI developers can’t entirely predict what a model will be like when they first start pre-training it.
“Any lab worth their salt has a whole team focused on leaderboards as part of post-training,” he said.
However Flynn also acknowledged that even this step isn’t good enough on its own.
He explained that his team has recorded discrepancies between how models rank on their red-teaming leaderboard, used to assess a model’s resistance to attacks such as prompt injection, and the risks those same models show when released into the real world.
“What we’ve found is that’s a good starting point but when you put it inside an application, when it has function-calling harnesses and there’s indirect attacks that are potentially possible, your synthetic test environment doesn’t always replicate what you’re seeing in the real world.
Urging all organizations to start using AI wherever they can, Rohrer added that he’s aware of the need for more hands-on support for leaders who don’t know how to evaluate AI models they’re looking to implement.
“You don’t have to learn how to train models to help in this space,” he said.
“I’m finding some of the best insights, for when I put data scientist and security folks together, is ‘Hey, we’re going to talk about this principle called control and data plane separation’ and the data scientist is like, ‘Well that’s not how the model works at all’ — and we’re like ‘That’s kind of a problem, let’s have a conversation’, even that is adding value.”
Coming together as an international community to share intelligence on these risks and form better methods for benchmarking complex AI systems will be key, the panel agreed.
“Internationally, there needs to be some baseline of consensus about what we’re actually talking about here, in terms of those capabilities and risks, and particularly when the risks are cross-border, and you can’t really do much about it in a given country,” said Leung.
AI threats and the evolving landscape
A recurring theme of RSAC Conference 2025 so far has been the evolving methods of attackers, particularly as they use AI to launch attacks more efficiently.
In response to a question on the likelihood of threat actors using AI to create polymorphic malware and other sophisticated code, Flynn acknowledged that AI models are becoming very good at writing in programming languages.
“In almost every respect that matters, this is really the year of coding,” Flynn said.
He argued that the current AI leaderboards, which measure coding across a range of benchmarks and assign models an average ‘ELO rating’, a term originally taken from chess, show publicly available models are becoming incredibly sophisticated at code generation.
“If you just look at the ELO scores on coding webbench or various types of leaderboards, you’ll see that there’s this unbelievable increase in the coding performance by the frontier models. And, as you can imagine, that has a knock-on effect towards being able to do types of things that you’re mentioning,” he said.
Flynn clarified that while he’s still unsure if it’s possible to achieve these kinds of attacks with AI, he predicts that we’ll know by the end of the year.
On even more theoretical grounds, the panel was divided over the degree to which artificial general intelligence (AGI) needs to be a consideration for security professionals at present. While Flynn was a co-author on a paper that predicted AGI could be created by 2030,
Rohrer argued that the date it arrives is less important than having the right framework to assess it when it does.
“What I’m really trying to understand is the divergence between my ability to measure the capabilities that are emergent as a collection, call it AGI, those capabilities and my ability to influence and control them,” he said.
“As long as those curves are moving together, I’m feeling pretty comfortable about any timeline.”
Source link