‘TPUs just work’: Why Google Cloud is betting big on its custom chips
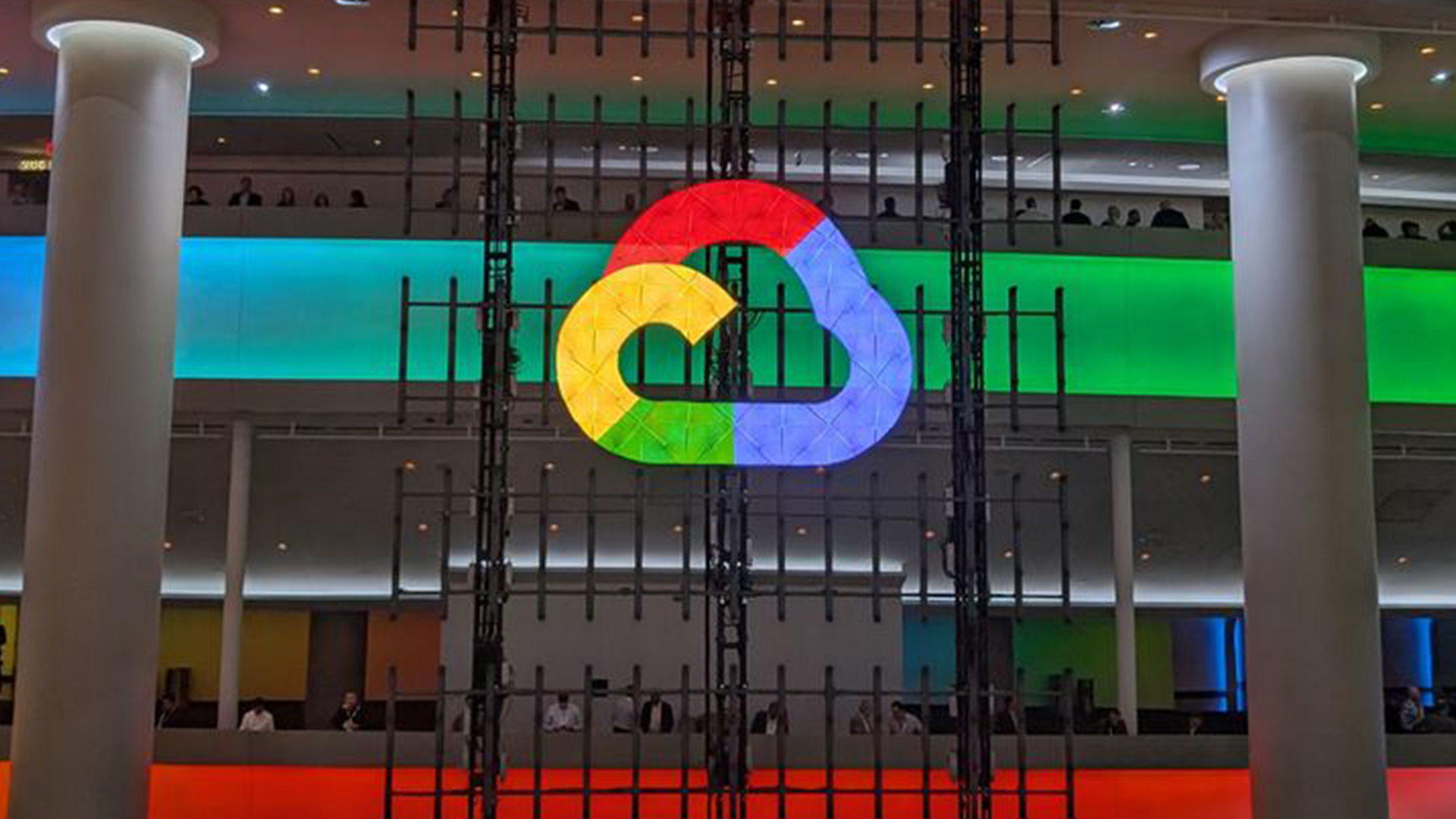
Google’s investment in tensor processing units (TPUs) is necessary to meet growing demand for AI inference, according to an expert at the firm.
At Google Cloud Next 2025, the cloud giant unveiled ‘Ironwood’, its seventh generation TPU, which it billed as a market-leading solution for running AI workloads at scale.
The chip will power Gemini and other AI models within Google Cloud and be made available to customers via the cloud for specialized AI training and inference purposes. Similarly, it competes with GPU offerings from rivals, with a promise of lower costs with no drop in performance.
Speaking to ITPro, George Elissaios, senior director of product management at Google, said the TPU has been “designed from the ground up as an AI platform”.
“We didn’t stumble into TPU, we were like ‘hey, can we build the best AI platform we can’, here are our requirements let’s go build it,” he said.
Elissaios pointed to the fact that from the very beginning, TPUs were designed from both a hardware and software perspective, with innovations like Google’s Pathways architecture for AI helping chips chain together for computing at scale.
Google Cloud unveiled its first generation TPU in 2018, and since then has been iterating on the technology to run its own AI services and those of its customers.
“TPUs just work, you can in a night start training at scale on TPUs, because the whole software stack has been battle tested for real applications” said Elissaios.
Google’s own models, including Gemini 2.5 Pro and Gemma 3, were trained on its TPUs. Anthropic also uses TPUs, having trained its Claude models with the infrastructure since Google’s fifth generation, per Data Center Dynamics.
Elissaios told ITPro that Google’s belief from the start was that customers would need a mix of GPU and TPU to meet AI training and inference demand as different models and workloads entered the market.
“You don’t ask yourself, ‘Why don’t we run SAP HANA on my laptop, why do we have a special system to run that?’,” he said.
AI will drive a similar need for investment in diverse hardware and specialized systems, Elissaios explained. Because the generative AI boom started with LLMs, some customers became fixated on the idea that LLM training was the primary workload and began to question the need for multiple compute solutions beyond GPUs.
“But we’re already at the stage where the workload is not just LLM training,” he added.
“We have LLM training, we have different types of LLM training, we have recommenders, we have inference, we have fine tuning. And then, on top of the diversity of the workloads, you have the diversity of architectures. Do I do an MoE, do I do a sparse model, is it a dense model?
“There are all of these things, is it a diffusion model, every day there is a DeepSeek model, every day there are new types and architectures.”
Add software stacks on top, which can lend themselves to specific model and architecture variants, and you have what Elissaios called “three or four different dimensions” that will shape an organization’s decision on which architecture to use.
“What we’re really focused on is helping customers that are now transitioning from training into inference, on building highly resilient services that they haven’t necessarily built before,” Elissaios said.
Changing approaches require hardware changes
To now, Elissaios explained, many Google Cloud customers have been focused on training models and consolidating data. Going forward, he believes customers will need to adopt a more operational mindset, with consideration for how to meet accelerated compute demand 24/7.
This is due to both increased use of AI tools and the adoption of AI agents, systems that run autonomously in the background.
Elissaios drew a stark contrast between running a web server connected to a company database, run on two machines, and running hundreds of GPUs that need to exchange data and scale to meet prompt demand.
This, he argued, is a strong reason to turn to a partner like Google which is used to running services at scale.
“Because guess what, when you’re building Gmail, YouTube, search, and ads, systems that are AI-first and serve multiple billions of people every day, with no exaggeration, you have that experience of how to build these systems, how to design them and that experience I can see it every day being transferred to our customers.”
Meeting intense demand
Ironwood is both Google Cloud’s most powerful and energy efficient chip, hailed by the company as a major step forward for AI infrastructure. Hundreds of thousands of Ironwood chips can be linked to work on AI training in tandem.
“The strain that any workload puts on the system, because we’re at the cutting edge, is incredible both in terms of heat, in terms of intensity to the network, and so on.”
Reducing this strain can come in the form of efficiency improvements – Ironwood offers double the flops per watt of its predecessor, for example – but also necessitate liquid cooling. This has the benefit of keeping chips at acceptable temperatures without expending too much energy but can also lead to massive freshwater wastage.
Elissaios told ITPro that water cooling can be the best solution, particularly in areas with no water access issues. He added that closed-loop water cooling systems can reduce water wastage and that emerging techniques such as using water evaporation to exchange heat are being pursued.
“The world is going to do AI, there’s no stopping that train,” he said. “Our responsibility is how do we, as providers [be] the most efficient, the best environmentally implemented solution for the world to do AI?”
Operational budgets
Inference is rising and Elissaios said this is shifting what was an R&D cost for businesses – training a model – to “cost of goods sold”.
“Every time I sell you an API call, my cost is the cost of running that inference,” he said. “That means everyone’s margins start depending on how efficiently I can do my inference.”
“And if you can do your inference efficiently enough, it almost doesn’t matter how much your training cost, because as long as there is volume, you can just spread the costs of training across billions, trillions of inference calls and you’re fine,” Elissaios added.
“So yes, smaller models do become very useful because when you don’t need to invoke a large model which is more expensive and you can get away by invoking a smaller model that helps the inference cost, that helps the margin, and makes a more sustainable business and a more sustainable industry.”
The differentiator for businesses, Elissaios said, is purely how costly it becomes to run the models and how this matches up with expectations. Budget doesn’t just apply to cost – it can also apply to the latency a business is willing to accept for an application or AI model and this is another area where small models can come in handy.
“That budget can go into network latency, it can go into model latency, data retrieval latency, and then you have to spread it out,” Elissaios explained.
This doesn’t mean that larger models are inherently slower to respond or more expensive. Google Cloud has invested significantly in improving its infrastructure to reduce latency across the board, and Elissaios told ITPro that through optimization some of the largest models available can still achieve competitive latencies.
“I think that in five years, ten years from now we’ll be looking back like ‘ok, that was just the beginning’. And of course, I can’t tell what the future is but that is the signal I’m getting from customers. It’s more customers building more models, more applications.”
MORE FROM ITPRO
Source link