Why AI researchers are turning to nature for inspiration
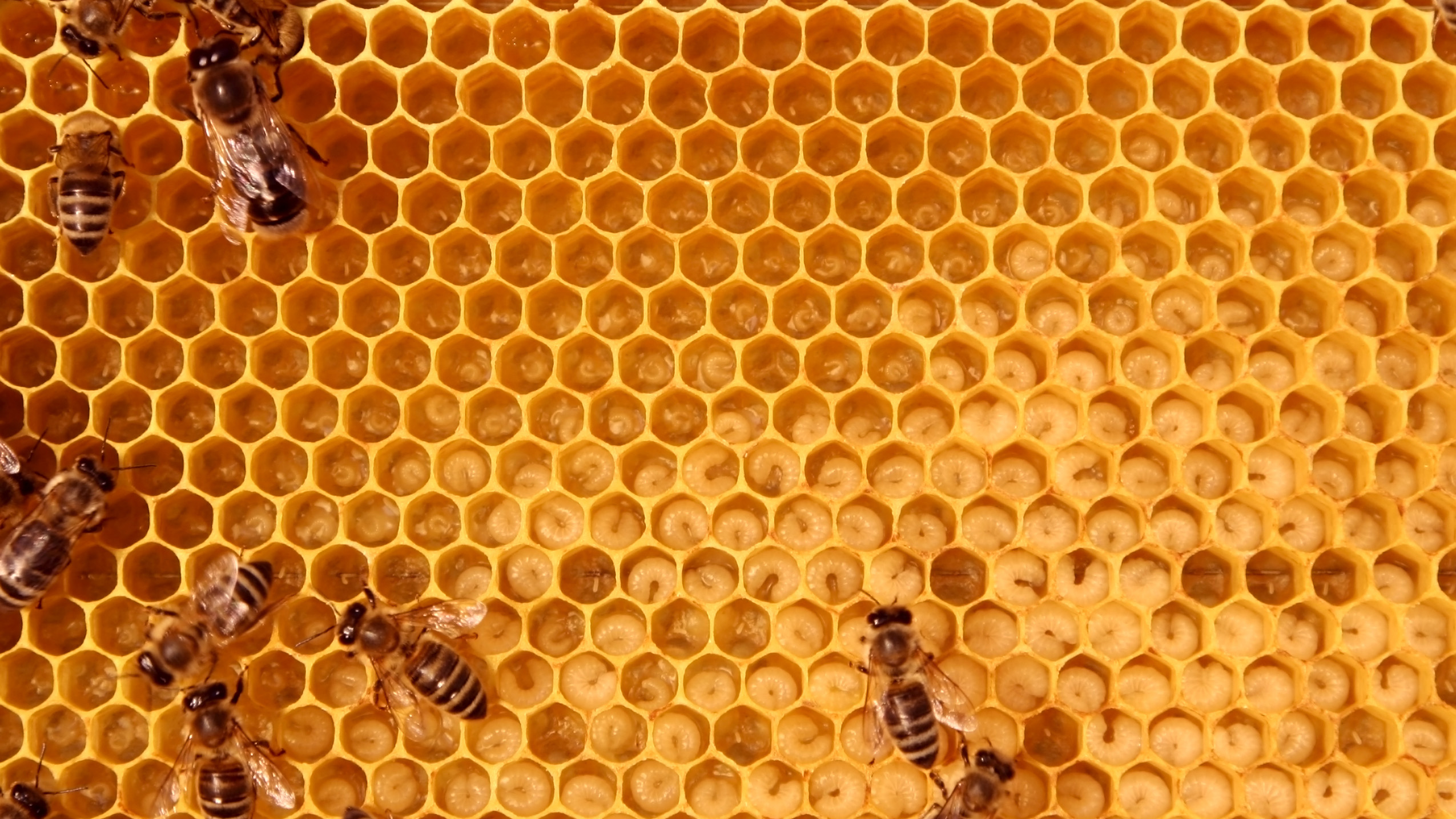
In the changing landscape of AI, researchers are increasingly turning to nature as a source of inspiration to overcome technological challenges and expand the limits of what machines are capable of achieving.
Technologies inspired by nature are also expanding. According to research from the Biomimicry Innovation Lab in collaboration with the Nadathur Group, patents for nature-inspired innovations have increased by 171% since 2010. The author Peter J. Bentley’s work is a good example; he investigates how digital systems could replicate human intelligence.
By studying natural systems, scientists are discovering new approaches to developing computing systems that are more efficient, adaptable, and capable of solving complex problems. For example, the intricate networks of neurons in the human brain are being studied to develop new neural networks and AI systems.
The collective behavior of bees has been mimicked by researchers at University College London to inform 3D printing drone activity, with similar work by the University of Sheffield feeding into potential delivery drones. Meanwhile, ant colony optimization algorithms replicate the collaborative behavior of these insects to optimize computing in a manner similar to agentic AI.
This trend is driven by a growing realization that many of the challenges traditional AI models face – such as efficiency, adaptability, and resilience – have already been solved by natural systems through millions of years of evolution. For example, natural selection has led to highly efficient systems for processing information, optimizing resources, and adapting to changing environments. By mimicking these systems, AI researchers hope to create more innovative, more sustainable, and more reliable technologies.
Nature’s decentralized and collective intelligence models, such as those observed in insect swarms or plant root systems, offer new paradigms for AI development. These systems function without a central controller, showcasing a degree of robustness and flexibility that centralized AI models often lack. This change in perspective encourages researchers to transition from monolithic AI models to more modular and adaptable systems capable of effectively managing the complexities of the real world.
Nature inspired AI: A diverse ecosystem
Nature has long been a blueprint for innovation, and recent advances in AI are taking this inspiration to new heights. From swarm intelligence to neuromorphic computing, nature-inspired methods are transforming the future of AI.
“In my mind, AI has always taken ideas from nature, whether its neural networks mimicking the brain, or evolutionary algorithms inspired by natural selection,” comments Cheney Hamilton, CEO at The Find Your Flex Group. “As AI research evolves, we’re seeing a shift beyond traditional models, with ideas from swarm intelligence, neuromorphic computing, and decentralized decision-making shaping the future of artificial intelligence.”
Swarm intelligence draws from the behavior of social insects like ants, bees, and birds, which demonstrate how simple agents following basic rules can create sophisticated solutions to complex problems. “Swarm-inspired systems provide a means to maintain diverse solutions and deliver robustness to unforeseen scenarios,” explains Mike Mangan, VP of Research at Opteran. He highlights how honeybees use democratic voting to make critical decisions, a natural principle that can enhance decision-making algorithms for fleets of autonomous robots.
Starling murmuration is one example of animals working together in a swam to achieve a beneficial outcome.
(Image credit: Getty Images)
Peter van der Putten, lead AI scientist at Pegasystems, also emphasizes the potential of swarm intelligence: “Many approaches to collective intelligence exist in AI, where smaller, simpler systems interact to solve tasks or display more complex higher-level behavior.” According to van der Putten, integrating generative AI into multi-agent systems transforms AI from a passive tool into a proactive agent capable of understanding problems, executing plans, and adapting to changing environments.
One ambitious initiative that showcases the integration of nature-inspired principles with AI development is the UK’s Advanced Research and Invention Agency (ARIA). This organization, described by the UK government as an executive, non departmental public body, aims to transform how computers process information by utilizing natural computation principles through its ‘Nature Computes Better’ project.
“From unravelling the basis of natural computation in single-celled organisms to demonstrating a commercially viable probabilistic processor, we’re funding an array of projects, with up to £500k each, across individual research teams, universities, and startups to maximize the chance of breakthroughs,” ARIA explains on its website.
This project emphasizes the potential of studying natural processes, such as the behavior of slime molds and the efficiency of plant root systems, to develop AI systems that are not only powerful but also energy-efficient and resilient.
Looking at human nature for efficient computing
Neuromorphic computing, which aims to replicate the human brain’s efficiency in processing information, is another field where nature-inspired principles are proving revolutionary.
IBM’s TrueNorth and Intel’s Loihi chips showcase how nature-inspired AI can enhance processing efficiency. These neuromorphic chips mimic the structure of biological brains by utilizing spiking neural networks, which process information how neurons communicate.
This brain-inspired approach offers significant energy efficiency and processing speed advantages, making these chips ideal for edge computing and low-power AI applications. As researchers continue to improve this technology, the potential for brain-like AI systems that learn and adapt with minimal energy consumption becomes more achievable.
“Neuromorphic processors have indeed come a long way in recent years, with compute clusters now containing billions of individually spiking neurons,” says Mangan. However, he cautions that while the technology is promising, it is still in its early days and faces challenges in achieving actual brain-like efficiency.
Brad Mallard, CTO at Version 1, adds that neuromorphic computing holds significant potential, “The biggest issue is real-time processing capacity and memory reference times needed for scalability.” He noted that while current systems may be more applicable to edge AI and smaller models, hardware miniaturization and parallel software development advances are needed before neuromorphic systems can become mainstream.
Evolutionary algorithms
Inspired by Darwinian evolution, evolutionary algorithms use principles of natural selection to evolve solutions over generations. These algorithms have been instrumental in optimization problems and AI training. “The approach taken in all major AI models is evolutionary in nature,” Version 1’s Mallard tells ITPro. “AI is designing its own neural networks, making models more efficient without human intervention and self-learning with AI models that develop and adapt without needing constant retraining.”
Van der Putten echoes this sentiment, noting that genetic programming and evolutionary algorithms can play a role in generative AI, synthetic data generation, and the design of new AI architectures.
But while nature offers powerful design principles, it also presents inherent limitations. As Hamilton points out, evolution is slow, messy, and full of compromises. Biological systems, while adaptable and efficient, often lack the precision and scalability required for advanced AI applications.
“Biology excels in adaptability and intuition but also has limitations—it tires and can misinterpret poorly defined tasks,” Mallard adds.
Ethical concerns can also arise as AI systems become more autonomous and decentralized. “Who’s responsible when a decentralized AI makes decisions no one understands?” asks Hamilton. She stresses the importance of maintaining human oversight, accountability, and transparency to prevent AI from adopting nature’s less desirable traits, such as unpredictability and competition.
The future of AI may involve a hybrid approach that fuses the most effective elements of nature-inspired designs with the benefits of synthetic technologies. By drawing inspiration from nature’s efficiency and adaptability, combined human-engineered innovations, AI researchers can create more innovative, safe, and scalable systems.
Nature continues to be a significant source of inspiration for AI research, providing solutions to complex problems beyond mere computational power. However, as researchers explore the natural world for insights, balancing this inspiration with caution is essential. They must ensure that AI systems remain safe, predictable, and aligned with human values.
Source link